“Using custom ML models, we predict the most effective in-app purchases”
Kseniia Maiboroda, CEO of Elevatix, talks ethics, creativity, and AI-driven personalisation for mobile games
Welcome to the second edition of AI Gamechangers, your newsletter where AI meets games. Each week, we’re sharing an interview with a leading games industry figure, plus a few links to relevant news. Forward this to your colleagues and encourage everyone to register for free - you won’t want to miss future editions (we have Q&As with the bosses of QuickSave, modl, Magicave and more already scheduled).
This week’s interview is with Kseniia Maiboroda of Elevatix. Scroll to the end for news on an AI project that simulates DOOM and OpenAI’s latest model.
Kseniia Maiboroda, Elevatix
In this AI|G feature, meet Kseniia Maiboroda, co-founder and CEO of Elevatix. Elevatix is revolutionising mobile game monetisation through AI-driven personalisation. The Elevatix machine learning [ML] engine works with mobile games, analysing player behaviour to create customised in-app purchase experiences in real time while adhering to strict privacy standards.
Top takeaways in this interview:
AI-powered monetisation works by analysing individual player behavior rather than using traditional cohort-based approaches.
In our "privacy first" world, such a behaviour-based approach deliberately avoids using personal data collection, so it works even with the 40% of players who don't consent to GDPR.
AI and ML are tools that can free up developers' creativity rather than replace it. By automating routine processes, studios can focus more time on creating new features and innovative gameplay.
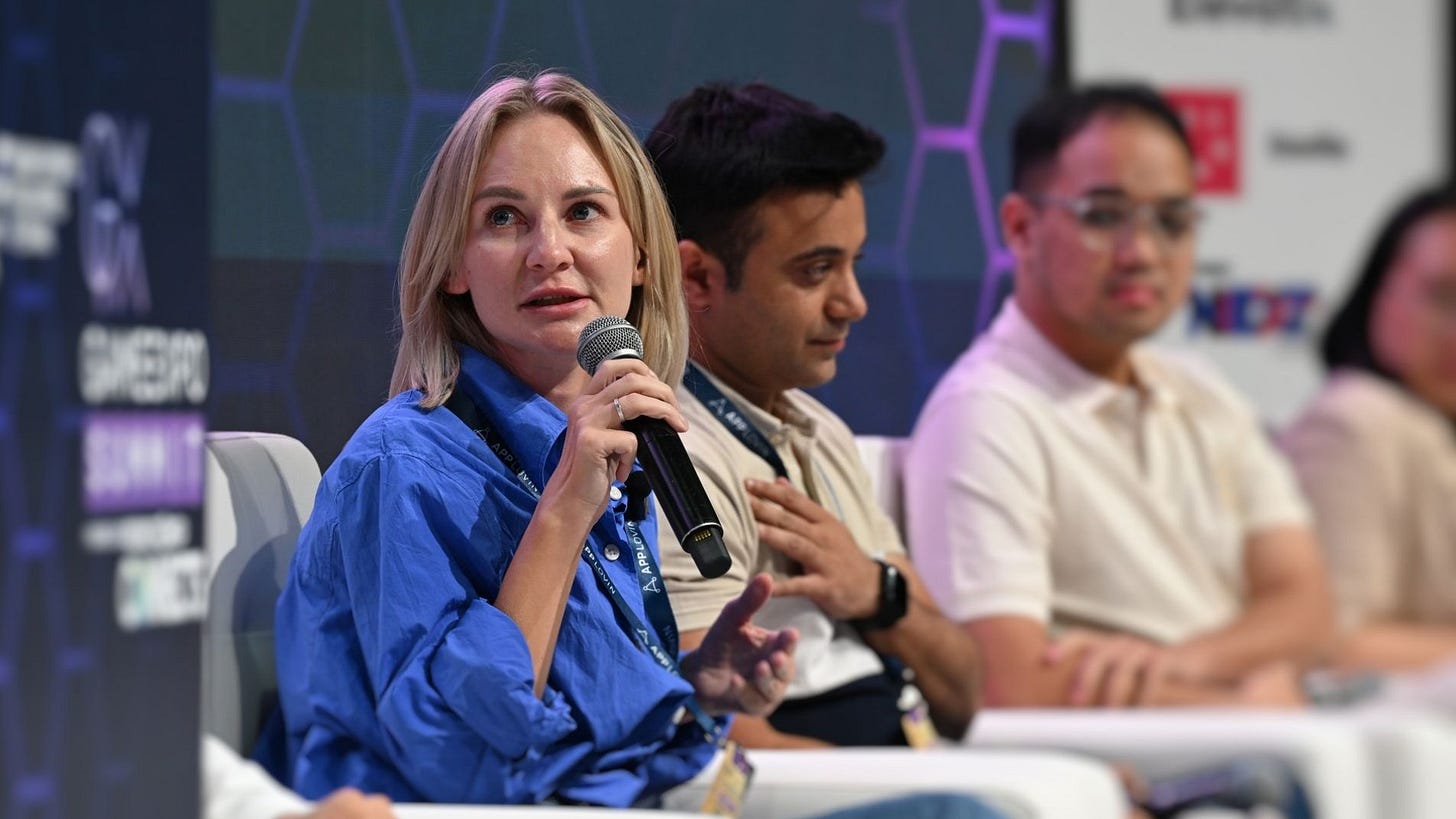
AI Gamechangers: Please tell us about Elevatix. What does it do?
Kseniia Maiboroda: It’s an engine that you can connect to any mobile game, by a very smooth process through the SDK (it takes a maximum of two days for one Unity developer). And with it, you’ll get your monetisation done in real-time.
We analyse the behaviour of each particular player in the game – we don’t use groups or cohorts or anything like that. Using our in-house, custom ML models, we make a prediction of the most effective in-app purchase chains for each player. This means we build a custom purchase flow and an IAP experience for each player. We make them feel it’s something very special for them. It’s unpredictable to them and a very different model from the general one currently used on the market.
When you have cohorts, and you set up similar in-app purchases for everyone in that cohort, players start to expect to see something at login, something when they level up, and so on. Because of that, the buying rate of these in-app purchases is mediocre, and not as effective as it can be. I see many games with fun gameplay, cool features, and so on, but the monetisation is not at the best level they could get. Often, players feel a little squeezed for money; they come to the game and get 10 in-app purchase offers at login and say, “I would like to play! I don’t want to close all these pop-ups first”. It’s very annoying. It ruins the retention rate. It ruins the user experience inside of the game.
So, we have created an absolutely different product. Our goal is to balance very effective monetisation for the game and a very comfortable and smooth user experience for the players. We want to give players the ability to enjoy the game and to buy what they want exactly in the place they like to do that, with a price that is comfortable for them.
Through ML, we customise the products you sell to players at a price that fits this particular player in the right place inside the game. We will show their personal offer at a frequency for each particular player because it can be very different even between two of us playing the same game because our behaviour inside the game is dissimilar.
Since your product personalises the experience, how do you ensure privacy? How can gamers know they’re not being spied on by AI?
It’s a great question because it’s the big issue now. We live in a “privacy first” world, so it’s very important to be aligned with all protocols: GDPR, CCPA, and so on.
Our approach is highly ethical, and we apply high ethical standards when creating our ML models and for the supervising of these ML models. We don’t use any personal player data, and we prove with our results that an “old style” approach (where you collect the player’s location, gender, age and all this stuff) is not as effective as when you track their behaviour. What does the player do in the game? If the player loses, what will they do then? If they win five levels in a row, what will they do? And so on and so on.
We don’t even take the player’s in-game ID. We take a “faceless” approach inside our ML models. We did it from scratch, so it’s a fully in-house solution. We don’t use any general models that you can find in other solutions. The general models are not bad. But as we know, in mobile gaming, if we look at the statistics in the United States, for example, more than 40% don’t consent to GDPR. They don’t want to share their personal data. If you try to align your personalisation approach with personal data, you will just lose this 40%! So you cannot make it like that. We understand this issue in the market, and we made a solution that has high ethical standards for the players on one side and, on the other side, is effective from a monetisation perspective for the games. So, we created custom algorithms that don’t use personal data.
How did you develop your in-house ML? Do you have a dedicated team working on it, and what were the principles?
We have everything in-house. Everyone on our team is from the mobile gaming industry. Everyone has a deep technical background in different ways.
I’m the author of the algorithms that we use for our ML. We have a CTO in our team who is really mature in ML, has a huge track record working with recommendation systems, and has worked for different startups and big companies. We have a dedicated ML engineer exactly for this domain. All of us are familiar with ML but from different perspectives. I’m more from maths and algorithms; our CTO is more from a technical perspective, the realisation of the combination system of the models and so on. We have a dedicated person who develops everything that we need, too. It’s the core technical expertise of our startup.
Please tell us a little bit about what metrics and player actions your system considers when it examines player behaviour. You mentioned it does not take personal data, but what is it considering?
I won’t give away the full secret recipe! But I can give you examples to just make it simple. It’s a topic [that comes up at] conferences, on blogs and so on. A lot of people who make games are a little bit sceptical. There are concerns that I often get from gaming producers because they’re not sure that the accuracy from machine learning or from artificial intelligence will be better than the accuracy done by people, by the liveops, and so on.
The second part they’re concerned about is the general idea that if you’d like to use ML, you have to provide a huge data set, right? I mean, an ML will not work without data. They think, “If I want to use ML, that means I have to wait half a year just to collect all this data, and only then will I get results.” I understand the situation because I come from mobile gaming and know how it works. From a business perspective, you don’t have time to wait six months to collect data to get some results. It’s the mobile gaming cycle: it’s impossible. Half a year is big money, you cannot wait.
We did our models in several layers. Our customers don’t have to wait [a long time] to collect data because we have really mature ML models that are supervised and studied exactly for mobile games. It’s not general models you might use for financial, insurance, or something else – it’s exactly for mobile gaming. And because of that, we don’t have the constraint that you have to wait to collect data sets; we already have really mature models that can give results very fast.
And the answer to your question about what behavioural parameters we usually look at? It is related to the genre of the game. So it will be different for social casino, for casual, for hypercasual. We have a general set that would be similar for everyone, too.
So let’s imagine that you and I are playing the same hypothetical game; my path in the game would be to play once a day, a 10-minute session, and that’s just my way. When I open the game, usually I start to play right after login, and then I play several levels in a row. Doesn’t matter if I lose or win, I just have 10 minutes, and I would like to play this session, maybe when I’m going through the subway to work. But for you, it will be absolutely different. So, for example, you open the game, and before you play the level, you’ll go into the shop, for example, or to a mini-game or to some features that you have, and only then do you start to play the levels - and you play until you lose. We analyse all these parameters: how often you play, how many sessions per day, what is your action first after the login, if the level is lost, will you continue trying to play this level, or will you just close the game, what is your action after a level won, after 10 levels in a row will you go to the shop to buy something, or will you just wait for the bonus in the next session?
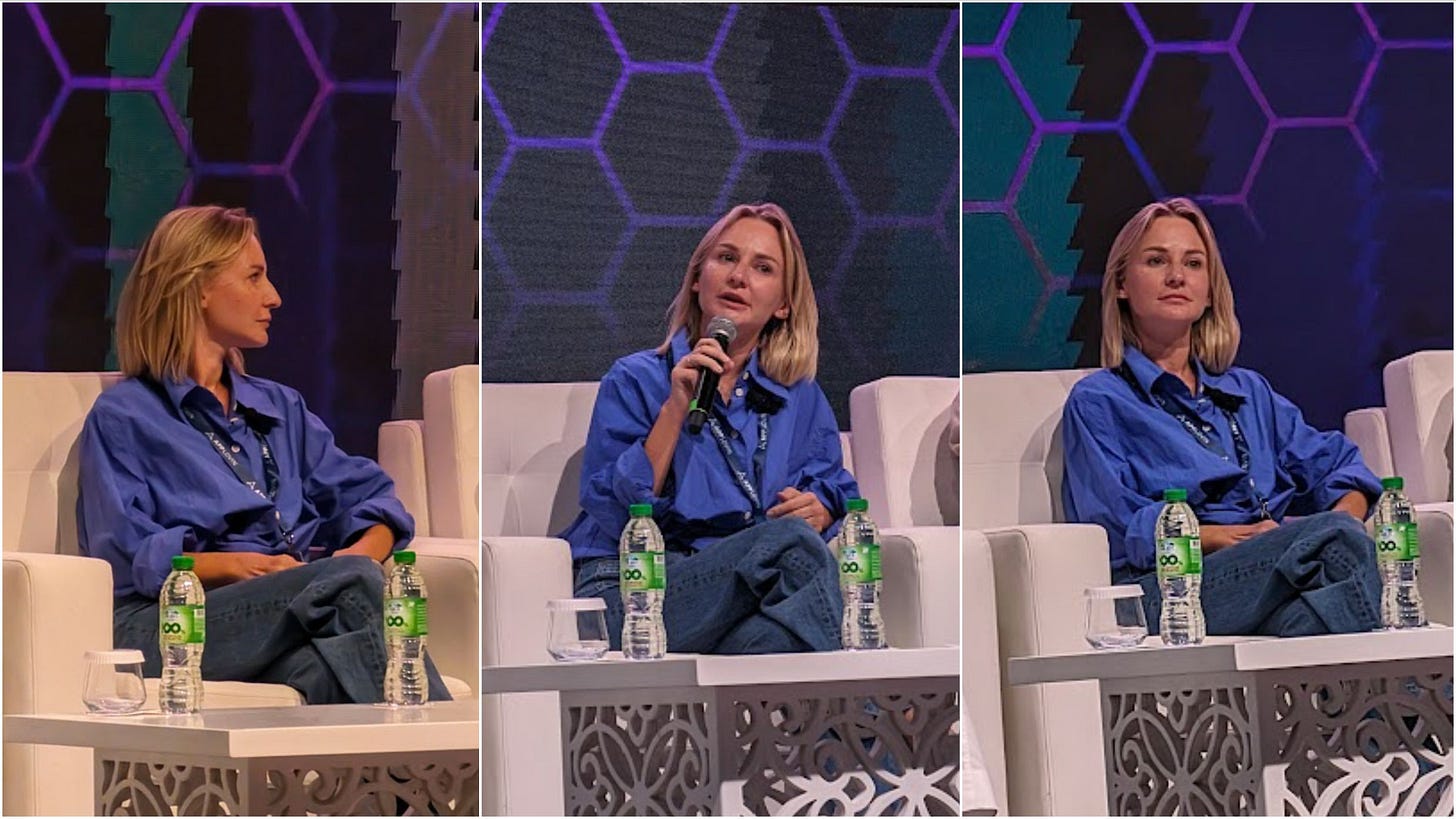
We analyse hundreds of parameters inside the game, and the real meaning is not just separate parameters. We analyse not only the action you take at one level (you lost the level, and you closed the game). No, we analyse the combination of everything that works before this action. We don’t just analyse your buying once a week, once a day. No, we analyse what was before that to build the next experience that will guide you. We analyse not just separate actions, and not just separate behavioural parameters; we analyse the chains of these parameters, and it provides us the ability to not only predict the most effective purchase for you but also when someone like you comes to the game and does similar actions to you, we already know what the right action is for this person! We’re not just personalising it for you; we can find similarities for new players.
In mobile games, it’s very important for the monetisation flow that you make conversions, because if you’re not making a good conversion, you just cannot get LTV [lifetime value] any more from this person. Making conversions is crucial for the ML because upscaling through the ML is not rocket science! If the person has already paid, it’s not rocket science to upscale to grow the LTV. However, making a conversion can be a big challenge because when a new player comes to the game, you don’t have data about that player. If you want to lean purely on personal data, it will not work in this case.
How do you see AI and machine learning evolving in the games industry in the next few years? What sense do you get that AI will be essential for many purposes?
I communicate a lot with people making games, not just from the technical side but also from the business side and from the creativity side. And I see tons of really bright ideas on the market. The blocker to scaling is the lack of technology.
[With AI], people will be able to concentrate on their creativity; they can brainstorm new cool features and new cool games, and tech can back them with their routine processes, like monetisation. Right now, they have high creativity, but they have to spend time finding strategies for monetisation, building liveops processes, scheduling all these events and more. It takes time, and competition in the gaming market now is tough. If you’d like to get good money from your game and be top-grossing in the stores, allow high tech to be your asset. You will win this battle because you will have much more time for creating new games and features, concentrating on your creativity, and innovating.
To create games, you don’t have to be an expert in ML – you have to be an expert in absolutely different domains. A lot of studios are trying to use AI to automate different processes. It doesn’t mean that you don’t need to have artists in your team! You need to have artists to create these concepts for sure. But for routine work, automating it through AI or ML is okay. This will give you more time to create the things where you are an expert, bringing your creative genius to the games.
What’s next for you and your company?
My big dream initially – and I still like following this dream – is to build a platform for mobile games. You won’t just make decisions for in-app purchase offers, as we have done already, but more. As I said previously, what will win in this big battle for the best mobile games? It’s you being creative and using technology as an asset. My goal is to make a platform where you will have different models that automate the processes you need for mobile games. You create a great game, then come to this platform, and with ML and artificial intelligence, you can connect all the stuff that can be automated in your development process. This way, you’ll save time, and you’ll get a great technical stack that will provide you with value to bring to your players.
Further down the rabbit hole
Some useful news, views and links to keep you going until next time…
OpenAI is reportedly prepping"Strawberry," a new AI model set to launch this autumn. It will excel at solving complex maths problems, developing marketing strategies, and tackling word puzzles (the example given is Connections in the New York Times), outperforming current models.
Battle of Three Kingdoms: double jump.tokyo partners with SEGA and Pictoria to launch a blockchain game featuring AI-powered character interactions. The game incorporates an AI Tuber, Da Qiao, for community management.
The upcoming PG Connects Helsinki conference, part of Finnish Games Week, features a Practical AI track. Tickets are available now (at a discounted Mid Term rate for a limited period). The event runs 1-2 October and speakers on AI include Harry Holmwood, Nasser Elmasri, Tomi Huttula and Oz Silahtar.
Google researchers have developed GameNGen, a neural model that can simulate DOOM in real time. Learning from gameplay recordings, it runs at 20+ fps using a diffusion model to predict frames based on previous actions, effectively functioning as a game engine. (Hat tip Professor Ethan Mollick.)